Compare commits
1 Commits
master
...
PYTHON_SKL
Author | SHA1 | Date | |
---|---|---|---|
![]() |
4e69ca18ef |
185
DataModels/GC.py
185
DataModels/GC.py
@ -2,26 +2,128 @@ from DataModels.Cell import Cell
|
|||||||
from DataModels.Road import Road
|
from DataModels.Road import Road
|
||||||
from DataModels.House import House
|
from DataModels.House import House
|
||||||
from DataModels.Dump import Dump
|
from DataModels.Dump import Dump
|
||||||
|
from DataModels.Grass import Grass
|
||||||
from config import GRID_WIDTH, GRID_HEIGHT, DELAY
|
from config import GRID_WIDTH, GRID_HEIGHT, DELAY
|
||||||
from utilities import movement, check_moves, save_moveset
|
from utilities import movement, check_moves, save_moveset, parse_coords
|
||||||
from Traversal.DFS import DFS
|
from Traversal.DFS import DFS
|
||||||
from Traversal.BestFS import BestFS
|
from Traversal.BestFS import BestFS
|
||||||
from Traversal.BFS import BFS
|
from Traversal.BFS import BFS
|
||||||
import pygame
|
import pygame
|
||||||
|
import numpy
|
||||||
|
from sklearn import svm, tree
|
||||||
|
import json
|
||||||
|
import pickle
|
||||||
|
|
||||||
|
MAPS = []
|
||||||
|
|
||||||
|
def map2int( objector ):
|
||||||
|
|
||||||
|
ci = type(objector)
|
||||||
|
|
||||||
|
if ci == Grass:
|
||||||
|
return 1
|
||||||
|
if ci == Road:
|
||||||
|
return 2
|
||||||
|
if ci == Dump:
|
||||||
|
return 3
|
||||||
|
|
||||||
|
if ci == House:
|
||||||
|
if objector.unvisited == True:
|
||||||
|
return 4
|
||||||
|
else:
|
||||||
|
return 1
|
||||||
|
|
||||||
|
def partial_map(grid, gc_pos, houseses):
|
||||||
|
grid = numpy.transpose(grid)
|
||||||
|
|
||||||
|
x,y = gc_pos[0],gc_pos[1]
|
||||||
|
map_part = []
|
||||||
|
|
||||||
|
#5x5
|
||||||
|
#coords = [ [x-2,y-2],[x-1,y-2],[x+0,y-2],[x+1,y-2],[x+2,y-2],
|
||||||
|
# [x-2,y-1],[x-1,y-1],[x+0,y-1],[x+1,y-1],[x+2,y-1],
|
||||||
|
# [x-2,y+0],[x-1,y+0],[x+0,y+0],[x+1,y+0],[x+2,y+0],
|
||||||
|
# [x-2,y+1],[x-1,y+1],[x+0,y+1],[x+1,y+1],[x+2,y+1],
|
||||||
|
# [x-2,y+2],[x-1,y+2],[x+0,y+2],[x+1,y+2],[x+2,y+2]]
|
||||||
|
|
||||||
|
#7x7
|
||||||
|
coords = [
|
||||||
|
[x-3,y-3],[x-2,y-3],[x-1,y-3],[x+0,y-3],[x+1,y-3],[x+2,y-3],[x+3,y-3],
|
||||||
|
[x-3,y-2],[x-2,y-2],[x-1,y-2],[x+0,y-2],[x+1,y-2],[x+2,y-2],[x+3,y-2],
|
||||||
|
[x-3,y-1],[x-2,y-1],[x-1,y-1],[x+0,y-1],[x+1,y-1],[x+2,y-1],[x+3,y-1],
|
||||||
|
[x-3,y+0],[x-2,y+0],[x-1,y+0],[x+0,y+0],[x+1,y+0],[x+2,y+0],[x+3,y+0],
|
||||||
|
[x-3,y+1],[x-2,y+1],[x-1,y+1],[x+0,y+1],[x+1,y+1],[x+2,y+1],[x+3,y+1],
|
||||||
|
[x-3,y+2],[x-2,y+2],[x-1,y+2],[x+0,y+2],[x+1,y+2],[x+2,y+2],[x+3,y+2],
|
||||||
|
[x-3,y+3],[x-2,y+3],[x-1,y+3],[x+0,y+3],[x+1,y+3],[x+2,y+3],[x+3,y+3]
|
||||||
|
]
|
||||||
|
|
||||||
|
for coord in coords:
|
||||||
|
flag = 0
|
||||||
|
|
||||||
|
for item in houseses:
|
||||||
|
if( coord == item ):
|
||||||
|
map_part.append(1)
|
||||||
|
flag = 1
|
||||||
|
break
|
||||||
|
|
||||||
|
if( flag == 1 ):
|
||||||
|
continue
|
||||||
|
|
||||||
|
if( 0 <= coord[1] < GRID_HEIGHT and 0 <= coord[0] < GRID_WIDTH ):
|
||||||
|
map_part.append(map2int(grid[coord[1]][coord[0]]))
|
||||||
|
else:
|
||||||
|
map_part.append(1)
|
||||||
|
|
||||||
|
return map_part
|
||||||
|
|
||||||
class GC(Cell):
|
class GC(Cell):
|
||||||
moves_made = 0
|
moves_made = 0
|
||||||
def __init__(self, x, y, max_rubbish, yellow=0, green=0, blue=0):
|
def __init__(self, x, y, max_rubbish, yellow=0, green=0, blue=0):
|
||||||
Cell.__init__(self, x, y, max_rubbish, yellow, green, blue)
|
Cell.__init__(self, x, y, max_rubbish, yellow, green, blue)
|
||||||
self.moves = []
|
self.moves = []
|
||||||
|
self.housesC = []
|
||||||
self.old_time = pygame.time.get_ticks()
|
self.old_time = pygame.time.get_ticks()
|
||||||
|
self.initial_position = [[x, y]]
|
||||||
|
self.run_training()
|
||||||
|
|
||||||
|
def run_trained(self, grid):
|
||||||
|
pos = [self.x,self.y]
|
||||||
|
print(partial_map(grid,pos,self.housesC))
|
||||||
|
direct = self.clf.predict([partial_map(grid,pos,self.housesC)])[0]
|
||||||
|
return direct
|
||||||
|
|
||||||
|
def run_training(self):
|
||||||
|
try:
|
||||||
|
with open('clf.json','rb') as fc:
|
||||||
|
self.clf = pickle.load(fc)
|
||||||
|
return
|
||||||
|
except:
|
||||||
|
pass
|
||||||
|
### SVM OR TREE
|
||||||
|
self.clf = svm.SVC(gamma='scale')
|
||||||
|
|
||||||
|
#self.clf = tree.DecisionTreeClassifier()
|
||||||
|
f = open('moveset_data.json','r')
|
||||||
|
datas = json.load(f)
|
||||||
|
|
||||||
|
X = []
|
||||||
|
y = []
|
||||||
|
|
||||||
|
for x in range(0,len(datas['moveset'])):
|
||||||
|
X += (datas['moveset'][x]['maps'])
|
||||||
|
|
||||||
|
for x in range(0,len(datas['moveset'])):
|
||||||
|
y += (datas['moveset'][x]['moves'])
|
||||||
|
|
||||||
|
self.clf.fit(X,y)
|
||||||
|
with open('clf.json','wb') as fc:
|
||||||
|
pickle.dump(self.clf,fc)
|
||||||
|
|
||||||
def move(self, direction, environment):
|
def move(self, direction, environment):
|
||||||
self.x, self.y = movement(environment, self.x, self.y)[0][direction]
|
self.x, self.y = movement(environment, self.x, self.y)[0][direction]
|
||||||
self.update_rect(self.x, self.y)
|
self.update_rect(self.x, self.y)
|
||||||
self.moves_made = self.moves_made + 1 #moves counter
|
self.moves_made = self.moves_made + 1 #moves counter
|
||||||
|
|
||||||
print(check_moves(environment, self.x, self.y,direction))
|
|
||||||
|
|
||||||
def collect(self, enviromnent):
|
def collect(self, enviromnent):
|
||||||
x, y = [self.x, self.y]
|
x, y = [self.x, self.y]
|
||||||
coordinates = [(x, y - 1), (x, y + 1), (x - 1, y), (x + 1, y)]
|
coordinates = [(x, y - 1), (x, y + 1), (x - 1, y), (x + 1, y)]
|
||||||
@ -34,6 +136,8 @@ class GC(Cell):
|
|||||||
continue
|
continue
|
||||||
|
|
||||||
if(type(item) == House or type(item) == Dump):
|
if(type(item) == House or type(item) == Dump):
|
||||||
|
if(type(item) == House):
|
||||||
|
self.housesC.append([item.x,item.y])
|
||||||
item.return_trash(self)
|
item.return_trash(self)
|
||||||
self.update_image()
|
self.update_image()
|
||||||
|
|
||||||
@ -56,6 +160,7 @@ class GC(Cell):
|
|||||||
self.moves.extend(result)
|
self.moves.extend(result)
|
||||||
element_list.append(house)
|
element_list.append(house)
|
||||||
|
|
||||||
|
|
||||||
for dump in range(dump_count):
|
for dump in range(dump_count):
|
||||||
avalible_moves = check_moves(enviromnent, x,y)
|
avalible_moves = check_moves(enviromnent, x,y)
|
||||||
if mode == "DFS":
|
if mode == "DFS":
|
||||||
@ -67,8 +172,43 @@ class GC(Cell):
|
|||||||
for x in element_list:
|
for x in element_list:
|
||||||
x.unvisited = True
|
x.unvisited = True
|
||||||
self.moves.reverse()
|
self.moves.reverse()
|
||||||
save_moveset(self.moves)
|
|
||||||
|
|
||||||
|
houseses = []
|
||||||
|
moves_L = (self.initial_position + (self.moves[::-1]))
|
||||||
|
last_pos = None
|
||||||
|
for i in range(len(moves_L)):
|
||||||
|
if( moves_L[i] == 'pick_garbage' ):
|
||||||
|
xc,yc = last_pos[0],last_pos[1]
|
||||||
|
|
||||||
|
possibl = []
|
||||||
|
|
||||||
|
if( xc-1 >= 0 ):
|
||||||
|
possibl.append([xc-1,yc])
|
||||||
|
if( xc+1 < GRID_WIDTH ):
|
||||||
|
possibl.append([xc+1,yc])
|
||||||
|
if( yc-1 >= 0 ):
|
||||||
|
possibl.append([xc,yc-1])
|
||||||
|
if( yc+1 < GRID_HEIGHT):
|
||||||
|
possibl.append([xc,yc+1])
|
||||||
|
#print("POSSIBL")
|
||||||
|
#print(possibl)
|
||||||
|
#print("##########")
|
||||||
|
for posi in possibl:
|
||||||
|
if( type(enviromnent[posi[0]][posi[1]]) == House ):
|
||||||
|
houseses.append(posi)
|
||||||
|
#MAPS.append(MAPS[-1])
|
||||||
|
MAPS.append(partial_map(enviromnent,last_pos,houseses) )
|
||||||
|
else:
|
||||||
|
last_pos = moves_L[i]
|
||||||
|
#print("HOUSES")
|
||||||
|
#print(houseses)
|
||||||
|
#print("##########")
|
||||||
|
MAPS.append(partial_map(enviromnent,moves_L[i],houseses) )
|
||||||
|
|
||||||
|
moves_to_file = []
|
||||||
|
maps_to_file = []
|
||||||
|
|
||||||
|
save_moveset(self.initial_position + (self.moves[::-1]), MAPS)
|
||||||
|
|
||||||
def find_houses_BestFS(self, environment):
|
def find_houses_BestFS(self, environment):
|
||||||
x = self.x
|
x = self.x
|
||||||
@ -103,8 +243,43 @@ class GC(Cell):
|
|||||||
[x,y],result,dump_list = output[0], output[1], output[2]
|
[x,y],result,dump_list = output[0], output[1], output[2]
|
||||||
self.moves.extend(result[1:])
|
self.moves.extend(result[1:])
|
||||||
self.moves.reverse()
|
self.moves.reverse()
|
||||||
save_moveset(self.moves)
|
|
||||||
|
|
||||||
|
houseses = []
|
||||||
|
moves_L = (self.initial_position + (self.moves[::-1]))
|
||||||
|
last_pos = None
|
||||||
|
for i in range(len(moves_L)):
|
||||||
|
if( moves_L[i] == 'pick_garbage' ):
|
||||||
|
xc,yc = last_pos[0],last_pos[1]
|
||||||
|
|
||||||
|
possibl = []
|
||||||
|
|
||||||
|
if( xc-1 >= 0 ):
|
||||||
|
possibl.append([xc-1,yc])
|
||||||
|
if( xc+1 < GRID_WIDTH ):
|
||||||
|
possibl.append([xc+1,yc])
|
||||||
|
if( yc-1 >= 0 ):
|
||||||
|
possibl.append([xc,yc-1])
|
||||||
|
if( yc+1 < GRID_HEIGHT):
|
||||||
|
possibl.append([xc,yc+1])
|
||||||
|
#print("POSSIBL")
|
||||||
|
#print(possibl)
|
||||||
|
#print("##########")
|
||||||
|
for posi in possibl:
|
||||||
|
if( type(environment[posi[0]][posi[1]]) == House ):
|
||||||
|
houseses.append(posi)
|
||||||
|
#MAPS.append(MAPS[-1])
|
||||||
|
MAPS.append(partial_map(environment,last_pos,houseses) )
|
||||||
|
else:
|
||||||
|
last_pos = moves_L[i]
|
||||||
|
#print("HOUSES")
|
||||||
|
#print(houseses)
|
||||||
|
#print("##########")
|
||||||
|
MAPS.append(partial_map(environment,moves_L[i],houseses) )
|
||||||
|
|
||||||
|
moves_to_file = []
|
||||||
|
maps_to_file = []
|
||||||
|
|
||||||
|
save_moveset(self.initial_position + (self.moves[::-1]), MAPS)
|
||||||
|
|
||||||
def make_actions_from_list(self,environment):
|
def make_actions_from_list(self,environment):
|
||||||
now = pygame.time.get_ticks()
|
now = pygame.time.get_ticks()
|
||||||
|
120
DataModels/GC.py.save
Normal file
120
DataModels/GC.py.save
Normal file
@ -0,0 +1,120 @@
|
|||||||
|
from DataModels.Cell import Cell
|
||||||
|
from DataModels.Road import Road
|
||||||
|
from DataModels.House import House
|
||||||
|
from DataModels.Dump import Dump
|
||||||
|
from config import GRID_WIDTH, GRID_HEIGHT, DELAY
|
||||||
|
from utilities import movement, check_moves, save_moveset
|
||||||
|
from Traversal.DFS import DFS
|
||||||
|
from Traversal.BestFS import BestFS
|
||||||
|
from Traversal.BFS import BFS
|
||||||
|
import pygame
|
||||||
|
|
||||||
|
class GC(Cell):
|
||||||
|
moves_made = 0
|
||||||
|
def __init__(self, x, y, max_rubbish, yellow=0, green=0, blue=0):
|
||||||
|
Cell.__init__(self, x, y, max_rubbish, yellow, green, blue)
|
||||||
|
self.moves = []
|
||||||
|
self.old_time = pygame.time.get_ticks()
|
||||||
|
def move(self, direction, environment):
|
||||||
|
self.x, self.y = movement(environment, self.x, self.y)[0][direction]
|
||||||
|
self.update_rect(self.x, self.y)
|
||||||
|
self.moves_made = self.moves_made + 1 #moves counter
|
||||||
|
|
||||||
|
print(check_moves(environment, self.x, self.y,direction))
|
||||||
|
|
||||||
|
def collect(self, enviromnent):
|
||||||
|
x, y = [self.x, self.y]
|
||||||
|
coordinates = [(x, y - 1), (x, y + 1), (x - 1, y), (x + 1, y)]
|
||||||
|
for coordinate in coordinates:
|
||||||
|
if coordinate[0]<0 or coordinate[1]<0:
|
||||||
|
continue
|
||||||
|
try:
|
||||||
|
item = enviromnent[coordinate[0]][coordinate[1]]
|
||||||
|
except:
|
||||||
|
continue
|
||||||
|
|
||||||
|
if(type(item) == House or type(item) == Dump):
|
||||||
|
item.return_trash(self)
|
||||||
|
self.update_image()
|
||||||
|
|
||||||
|
def get_moves_count(self):
|
||||||
|
return self.moves_made
|
||||||
|
|
||||||
|
def find_houses(self,enviromnent, house_count,dump_count, mode):
|
||||||
|
x = self.x
|
||||||
|
y = self.y
|
||||||
|
result = []
|
||||||
|
element_list=[]
|
||||||
|
house_count_after_search=house_count
|
||||||
|
for home in range(house_count):
|
||||||
|
avalible_moves = check_moves(enviromnent, x,y)
|
||||||
|
if mode == "DFS":
|
||||||
|
house,[x,y],result = DFS(enviromnent,avalible_moves,[[x,y]],House)
|
||||||
|
elif mode == "BFS":
|
||||||
|
house,[x,y],result = BFS(enviromnent,avalible_moves,[[x,y]],House)
|
||||||
|
result = result[1::]
|
||||||
|
self.moves.extend(result)
|
||||||
|
element_list.append(house)
|
||||||
|
|
||||||
|
for dump in range(dump_count):
|
||||||
|
avalible_moves = check_moves(enviromnent, x,y)
|
||||||
|
if mode == "DFS":
|
||||||
|
dump,[x,y],result = DFS(enviromnent,avalible_moves,[[x,y]],Dump)
|
||||||
|
elif mode == "BFS":
|
||||||
|
dump,[x,y],result = BFS(enviromnent,avalible_moves,[[x,y]],Dump)
|
||||||
|
self.moves.extend(result)
|
||||||
|
element_list.append(dump)
|
||||||
|
for x in element_list:
|
||||||
|
x.unvisited = True
|
||||||
|
self.moves.reverse()
|
||||||
|
save_moveset(self.moves)
|
||||||
|
|
||||||
|
|
||||||
|
def find_houses_BestFS(self, environment):
|
||||||
|
x = self.x
|
||||||
|
y = self.y
|
||||||
|
result = [[x,y]]
|
||||||
|
|
||||||
|
houses_list = []
|
||||||
|
dump_list = []
|
||||||
|
a = 0
|
||||||
|
for row in environment:
|
||||||
|
b = 0
|
||||||
|
for col in row:
|
||||||
|
if (type(col) is House):
|
||||||
|
houses_list.append([col,[a,b]])
|
||||||
|
if (type(col) is Dump):
|
||||||
|
dump_list.append([col,[a,b]])
|
||||||
|
b += 1
|
||||||
|
a += 1
|
||||||
|
|
||||||
|
x, y = self.x, self.y
|
||||||
|
|
||||||
|
for i in range(len(houses_list)):
|
||||||
|
available_movement = check_moves(environment, x, y)
|
||||||
|
output = BestFS(environment, available_movement, [[x,y]], houses_list)
|
||||||
|
if(output != None):
|
||||||
|
[x,y],result,houses_list = output[0], output[1], output[2]
|
||||||
|
self.moves.extend(result[1:])
|
||||||
|
for i in range(len(dump_list)):
|
||||||
|
available_movement = check_moves(environment, x, y)
|
||||||
|
output = BestFS(environment, available_movement, [[x,y]], dump_list)
|
||||||
|
if(output != None):
|
||||||
|
[x,y],result,dump_list = output[0], output[1], output[2]
|
||||||
|
self.moves.extend(result[1:])
|
||||||
|
self.moves.reverse()
|
||||||
|
save_moveset(self.moves)
|
||||||
|
|
||||||
|
|
||||||
|
def make_actions_from_list(self,environment):
|
||||||
|
now = pygame.time.get_ticks()
|
||||||
|
if len(self.moves)==0 or now - self.old_time <= DELAY:
|
||||||
|
return
|
||||||
|
self.old_time = pygame.time.get_ticks()
|
||||||
|
if self.moves[-1] == "pick_garbage":
|
||||||
|
self.collect(environment)
|
||||||
|
self.moves.pop()
|
||||||
|
return
|
||||||
|
self.x, self.y = self.moves.pop()
|
||||||
|
self.moves_made = self.moves_made + 1 #moves counter
|
||||||
|
self.update_rect(self.x,self.y)
|
@ -4,6 +4,8 @@ def GenerateMap():
|
|||||||
#generate random empty map
|
#generate random empty map
|
||||||
width = random.randint(5,15) #up to 15
|
width = random.randint(5,15) #up to 15
|
||||||
height = random.randint(5,10) #up to 10
|
height = random.randint(5,10) #up to 10
|
||||||
|
width = 10
|
||||||
|
height = 10
|
||||||
grid = []
|
grid = []
|
||||||
|
|
||||||
row = []
|
row = []
|
||||||
|
@ -1,89 +0,0 @@
|
|||||||
---
|
|
||||||
|
|
||||||
# SVM raport
|
|
||||||
|
|
||||||
##### Konrad Pierzyński
|
|
||||||
|
|
||||||
###### Śmieciarz
|
|
||||||
|
|
||||||
12.06.2019
|
|
||||||
|
|
||||||
---
|
|
||||||
|
|
||||||
**SVM** - **S**upport-**V**ector **M**achine - zestaw metod uczenia stosowanych głównie do klasyfikacji, której nauka ma na celu wyznaczenie płaszczyzn rozdzielających dane wejściowe na klasy.
|
|
||||||
|
|
||||||
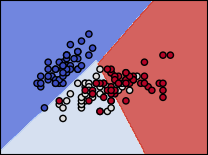
|
|
||||||
|
|
||||||
---
|
|
||||||
|
|
||||||
### Przygotowanie danych
|
|
||||||
|
|
||||||
Dane uczące zostały wygenerowane w następujący sposób:
|
|
||||||
|
|
||||||
+ Program generuje losową mapę o określonych wymiarach
|
|
||||||
|
|
||||||
+ Uruchamiany jest jeden z algorytmów (*BestFirstSearch*), który generuje listę ruchów.
|
|
||||||
|
|
||||||
+ Do zestawu uczącego dopisywana jest para składająca się na ruch i otoczenie gracza.
|
|
||||||
|
|
||||||
- Ruch odpowiada kierunkom: góra, prawo, dół, lewo i akcji zebrania/oddania śmieci - odpowienio liczbowo 1, 2, 3, 4, 99
|
|
||||||
|
|
||||||
- Otocznie to tablica dwuwymiarowa 7x7, gdzie element środkowy to pozycja gracza. Tablica ta następnie spłaszczana jest do tablicy jednowymiarowej
|
|
||||||
|
|
||||||
- Każdy 'domek', na którym została wykonana już akcja zebrania i jest opróżniony, widoczny jest na mapie tak samo jak element otoczenia, z którym gracz nie może wejść w żadną interakcję (stanąć, zebrać)
|
|
||||||
|
|
||||||
- Jeśli siatka 7x7 wykracza swoim zakresem za mapę, siatka uzupełniana jest przez trawę, czyli obiekt, z którym gracz nie wchodzi w interakcję
|
|
||||||
|
|
||||||
+ Po przejściu całej mapy algorytmem i zebraniu danych proces jest powtarzany tak długo, by zgromadzić około tysiąc rozwiązanych map
|
|
||||||
|
|
||||||
Pojedynczy zestaw danych jest zapisywany jako json postaci:
|
|
||||||
|
|
||||||
```json
|
|
||||||
{
|
|
||||||
"maps": [
|
|
||||||
[Int, Int, ...],
|
|
||||||
[Int, Int, ...],
|
|
||||||
...
|
|
||||||
],
|
|
||||||
"moves":
|
|
||||||
[
|
|
||||||
Int, Int, ...
|
|
||||||
]
|
|
||||||
}
|
|
||||||
```
|
|
||||||
|
|
||||||
I dopisywany do głównej struktury:
|
|
||||||
|
|
||||||
```json
|
|
||||||
{
|
|
||||||
"moveset": [
|
|
||||||
Zestaw, Zestaw, ...
|
|
||||||
]
|
|
||||||
}
|
|
||||||
```
|
|
||||||
|
|
||||||
---
|
|
||||||
|
|
||||||
### Uczenie
|
|
||||||
|
|
||||||
Do przeprowadzenia procesu uczenia dane uczące zostały podzielone na dwie listy:
|
|
||||||
|
|
||||||
- Pierwsza lista X zawiera wszystkie mapy częściowe (otoczenia)
|
|
||||||
|
|
||||||
```X = [ [Int, Int, ...], [Int, Int, ...], ... ]```
|
|
||||||
|
|
||||||
- Druga lista y zawiera odpowiadające mapom ruchy (1,2,3,4,99), które wykonał algorytm (*BestFirstSearch*) na danych otoczeniach.
|
|
||||||
|
|
||||||
```y = [ Int, Int, ... ]```
|
|
||||||
|
|
||||||
Wyżej wymienione dwie listy zostały podane jako argument metodzie ```fit(X,y)```, która odpowiada za uczenie się SVM. Natomiast utworzenie samego obiektu polega na zaimportowaniu biblioteki *scikit-learn*:
|
|
||||||
```from sklearn import svm```
|
|
||||||
a następnie już same utworzenia obiektu svm:
|
|
||||||
```clf = svm.SVC(gamma='scale')```
|
|
||||||
Wyuczony obiekt jest zapisywany do pliku, dzięki modułowi ```pickle```, aby nie przeprowadzać procesu uczenia za każdym uruchomieniem programu.
|
|
||||||
|
|
||||||
---
|
|
||||||
|
|
||||||
### Wykonywanie ruchów
|
|
||||||
|
|
||||||
Do przewidywania ruchów wystarczy użyć metody ```predict([ [otoczenie] ])``` , które przyjmuje mapę częściową, a jej wynik jest akcją, którą powinien wykonać gracz. Wynik metody przekazywany jest graczowi, który wykonuje ruch.
|
|
@ -1,92 +0,0 @@
|
|||||||
# Sztuczna Inteligencja 2019 - Raport Indywidualny
|
|
||||||
|
|
||||||
**Czas trwania opisywanych prac:** 09.05.2019 - 11.06.2019
|
|
||||||
|
|
||||||
**Autor:** Michał Starski
|
|
||||||
|
|
||||||
**Wybrany temat:** Inteligentna śmieciarka
|
|
||||||
|
|
||||||
**Link do repozytorium projektu:** https://git.wmi.amu.edu.pl/s440556/SZI2019SmieciarzWmi
|
|
||||||
|
|
||||||
## Wybrany algorytm uczenia - drzewa decyzyjne
|
|
||||||
|
|
||||||
### Przygotowane dane
|
|
||||||
|
|
||||||
Aby zapewnić smieciarce jak najlepszy wynik, do przygotowania danych do uczenia wybrałem algorytm szukania najkrótszej ścieżki, który dawał najlepsze wyniki podczas projektu grupowego - **BestFS**.
|
|
||||||
|
|
||||||
Podczas każdego jednorazowego przebiegu algorytmu BestFS patrzyłem na to jaki krok śmieciarka wykonuje w danej sytuacji, a następnie dane kroki zapisywałem do pliku w formacie json, tworząc próbki do późniejszej nauki.
|
|
||||||
|
|
||||||
Przykładowa próbka w formacie json:
|
|
||||||
|
|
||||||
```json
|
|
||||||
{
|
|
||||||
"moveset": [
|
|
||||||
{
|
|
||||||
"maps": [[1, 1, 3, 4, 2, 2, 2, 2, 1], [2, 1, 1, 3, 1, 4, 1, 1, 1]],
|
|
||||||
"moves": [1, 2]
|
|
||||||
}
|
|
||||||
]
|
|
||||||
}
|
|
||||||
```
|
|
||||||
|
|
||||||
`moveset` to tablica wszystkich próbek wykorzystywanych do nauki.
|
|
||||||
Każdy element tablicy to obiekt posiadający dwa pola:
|
|
||||||
`maps` - otoczenie śmieciarki w danym kroku,
|
|
||||||
`moves` - ruch śmieciarki przy danym otoczeniu
|
|
||||||
|
|
||||||
W powyższym przykładzie dla czytelności, zostały przedstawione otoczenia 3x3 wokół śmieciarki. W implementacji obszar ten został powiększony do 7x7 w celu poprawienia dokładności algorytmu.
|
|
||||||
|
|
||||||
#### Maps
|
|
||||||
|
|
||||||
Spłaszczona tablicę dwuwymiarową przedstawiająca otoczenie śmieciarki w konkretnym momencie działania algorytmu. Każda z cyfr przedstawia inny obiekt na mapie:
|
|
||||||
|
|
||||||
- 1 - Trawa (Grass)
|
|
||||||
- 2 - Droga (Road)
|
|
||||||
- 3 - Wysypisko (Dump)
|
|
||||||
- 4 - Dom (House)
|
|
||||||
|
|
||||||
Dla powyższego przykładu pierwsza sytuacja (`moveset[0].maps[0]`) przedstawia następujące otoczenie na mapie
|
|
||||||
|
|
||||||
```
|
|
||||||
G G D
|
|
||||||
H R R
|
|
||||||
R R G
|
|
||||||
```
|
|
||||||
|
|
||||||
#### Moves
|
|
||||||
|
|
||||||
Tablica ruchów śmieciarki. i-ty ruch w tablicy odpowiada i-temu otoczeniu. Wyróżnimay 5 różnych ruchów agenta:
|
|
||||||
|
|
||||||
- 1 - Lewo
|
|
||||||
- 2 - Prawo
|
|
||||||
- 3 - Dół
|
|
||||||
- 4 - Góra
|
|
||||||
- 99 - Zbierz śmieci
|
|
||||||
|
|
||||||
Tak więc dla powyższego otoczenia `1` będzie oznaczać, że agent ruszył się w lewo.
|
|
||||||
|
|
||||||
---
|
|
||||||
|
|
||||||
### Implementacja
|
|
||||||
|
|
||||||
Do implementacji uczenia poprzez drzewo decyzyjny wykorzystałem bibliotekę [scikit learn](https://scikit-learn.org) do języka **python**. Podając odpowiednie dane, biblioteka przygotuje nam model zdolny do samodzielnego poruszania się na mapie.
|
|
||||||
|
|
||||||
```python
|
|
||||||
#Trenowanie modelu
|
|
||||||
from sklearn import tree
|
|
||||||
X = [Kolejne otoczenia 7x7 w danym kroku]
|
|
||||||
Y = [Kolejne kroki odpowiednie dla danego otoczenia]
|
|
||||||
clf = tree.DecisionTreeClassifier()
|
|
||||||
clf = clf.fit(X, Y)
|
|
||||||
|
|
||||||
#Samodzielny ruch wytrenowanego modelu
|
|
||||||
clf.predict([Otoczenie agenta])
|
|
||||||
```
|
|
||||||
|
|
||||||
`clf.predict` zwróci nam 1 z 5 ruchów, które ma wykonać agent.
|
|
||||||
|
|
||||||
---
|
|
||||||
|
|
||||||
### Obserwacje
|
|
||||||
|
|
||||||
W idealnym przypadku wytrenowany model powinien odzwierciedlać algorytm BestFS, jako iż to na podstawie jego był trenowany i to jego decyzje starał się naśladować. W rzeczywistości jednak po przygotowaniu ok. 1000 próbek agent radził sobie różnorako. Na jednych mapach poruszał się dość sprawnie, jednak na wielu nie wiedział co ma robić. Przyczyny mogą być różne, jednak w mojej opinii, przygotowanych danych było jednak trochę za mało i gdyby dać o wiele więcej danych do wytrenowania modelu, rezultat byłby o wiele lepszy.
|
|
@ -1,6 +1,8 @@
|
|||||||
from utilities import movement,check_moves
|
from utilities import movement,check_moves
|
||||||
from DataModels.House import House
|
from DataModels.House import House
|
||||||
from DataModels.Dump import Dump
|
from DataModels.Dump import Dump
|
||||||
|
from DataModels.Grass import Grass
|
||||||
|
from DataModels.Road import Road
|
||||||
from DataModels.Container import Container
|
from DataModels.Container import Container
|
||||||
from config import GRID_WIDTH, GRID_HEIGHT
|
from config import GRID_WIDTH, GRID_HEIGHT
|
||||||
|
|
||||||
@ -14,6 +16,7 @@ def DFS(grid, available_movement, gc_moveset, mode,depth=0):
|
|||||||
possible_goals.append([a,b+1])
|
possible_goals.append([a,b+1])
|
||||||
possible_goals.append([a,b-1])
|
possible_goals.append([a,b-1])
|
||||||
object_in_area = False
|
object_in_area = False
|
||||||
|
|
||||||
for location in possible_goals:
|
for location in possible_goals:
|
||||||
if GRID_WIDTH>location[0]>=0 and GRID_HEIGHT>location[1]>=0:
|
if GRID_WIDTH>location[0]>=0 and GRID_HEIGHT>location[1]>=0:
|
||||||
cell = grid[location[0]][location[1]]
|
cell = grid[location[0]][location[1]]
|
||||||
@ -29,11 +32,13 @@ def DFS(grid, available_movement, gc_moveset, mode,depth=0):
|
|||||||
|
|
||||||
if len(available_movement) == 0 or depth>30:
|
if len(available_movement) == 0 or depth>30:
|
||||||
return
|
return
|
||||||
|
|
||||||
for direction in available_movement:
|
for direction in available_movement:
|
||||||
x_next, y_next = movement(grid,x,y)[0][direction]
|
x_next, y_next = movement(grid,x,y)[0][direction]
|
||||||
available_movement_next = check_moves(grid, x_next,y_next,direction)
|
available_movement_next = check_moves(grid, x_next,y_next,direction)
|
||||||
gc_moveset_next = gc_moveset.copy()
|
gc_moveset_next = gc_moveset.copy()
|
||||||
gc_moveset_next.append([x_next,y_next])
|
gc_moveset_next.append([x_next,y_next])
|
||||||
|
|
||||||
result = DFS(grid, available_movement_next, gc_moveset_next,mode, depth+1)
|
result = DFS(grid, available_movement_next, gc_moveset_next,mode, depth+1)
|
||||||
if result!= None:
|
if result!= None:
|
||||||
return result
|
return result
|
||||||
|
@ -3,7 +3,7 @@ from MapGenerator import GenerateMap
|
|||||||
|
|
||||||
CELL_SIZE = 64
|
CELL_SIZE = 64
|
||||||
FPS = 60
|
FPS = 60
|
||||||
DELAY = 50
|
DELAY = 250
|
||||||
|
|
||||||
try:
|
try:
|
||||||
MAP_NAME = sys.argv[1]
|
MAP_NAME = sys.argv[1]
|
||||||
|
13
main.py
13
main.py
@ -81,6 +81,12 @@ gc = GC(GC_X, GC_Y, 200)
|
|||||||
print("GC: " + str(GC_X) + str(GC_Y))
|
print("GC: " + str(GC_X) + str(GC_Y))
|
||||||
pygame_sprites.add(gc)
|
pygame_sprites.add(gc)
|
||||||
|
|
||||||
|
#gc.find_houses_BestFS(map_objects)
|
||||||
|
#pygame.quit()
|
||||||
|
#sys.exit()
|
||||||
|
|
||||||
|
svmmv = {1: "up", 2: "right", 3:"down",4:"left"}
|
||||||
|
|
||||||
while True:
|
while True:
|
||||||
|
|
||||||
for event in pygame.event.get():
|
for event in pygame.event.get():
|
||||||
@ -104,6 +110,13 @@ while True:
|
|||||||
gc.find_houses_BestFS(map_objects)
|
gc.find_houses_BestFS(map_objects)
|
||||||
elif event.key == pygame.K_8:
|
elif event.key == pygame.K_8:
|
||||||
gc.find_houses(map_objects,house_count,dump_count, "BFS")
|
gc.find_houses(map_objects,house_count,dump_count, "BFS")
|
||||||
|
elif event.key == pygame.K_n:
|
||||||
|
ppp = gc.run_trained(map_objects)
|
||||||
|
print(ppp)
|
||||||
|
if( ppp == 99 ):
|
||||||
|
gc.collect(map_objects)
|
||||||
|
else:
|
||||||
|
gc.move(svmmv[ppp], map_objects)
|
||||||
|
|
||||||
gc.make_actions_from_list(map_objects)
|
gc.make_actions_from_list(map_objects)
|
||||||
pygame_sprites.update()
|
pygame_sprites.update()
|
||||||
|
@ -1,2 +1,4 @@
|
|||||||
Pillow==5.4.1
|
Pillow==5.4.1
|
||||||
pygame==1.9.5
|
pygame==1.9.5
|
||||||
|
numpy
|
||||||
|
scikit-learn
|
7
run.bat
Normal file
7
run.bat
Normal file
@ -0,0 +1,7 @@
|
|||||||
|
@echo off
|
||||||
|
|
||||||
|
:1
|
||||||
|
start /W WENV\Scripts\python.exe main.py
|
||||||
|
goto 1
|
||||||
|
|
||||||
|
pause
|
54
utilities.py
54
utilities.py
@ -24,7 +24,45 @@ def check_moves(environment, x,y,direction=None):
|
|||||||
return ([dir for dir in movement(environment, x, y)[0] if movement(environment, x,y)[0][dir] != (x,y)])
|
return ([dir for dir in movement(environment, x, y)[0] if movement(environment, x,y)[0][dir] != (x,y)])
|
||||||
return ([dir for dir in movement(environment, x, y)[0] if movement(environment, x,y)[0][dir] != (x,y) and dir != movement(environment,x,y)[1][direction]])
|
return ([dir for dir in movement(environment, x, y)[0] if movement(environment, x,y)[0][dir] != (x,y) and dir != movement(environment,x,y)[1][direction]])
|
||||||
|
|
||||||
def save_moveset(moveset):
|
def define_dir(coord1, coord2):
|
||||||
|
if( coord1[0]-coord2[0] > 0):
|
||||||
|
return 4
|
||||||
|
elif( coord1[0]-coord2[0] < 0 ):
|
||||||
|
return 2
|
||||||
|
elif( coord1[0]-coord2[0] == 0):
|
||||||
|
if( coord1[1]-coord2[1] > 0):
|
||||||
|
return 1
|
||||||
|
elif( coord1[1]-coord2[1] < 0):
|
||||||
|
return 3
|
||||||
|
else:
|
||||||
|
return -1
|
||||||
|
|
||||||
|
def parse_coords(coords):
|
||||||
|
|
||||||
|
#left = 4 right = 2 up = 1 down = 3 pick_garbage = 99
|
||||||
|
crd = list(coords)
|
||||||
|
output = []
|
||||||
|
|
||||||
|
#for i in range(0,len(crd)-1):
|
||||||
|
# while( crd[i+1] == "pick_garbage" ):
|
||||||
|
# crd.pop(i+1)
|
||||||
|
# output.append(99)
|
||||||
|
# output.append(define_dir(crd[i],crd[i+1]))
|
||||||
|
|
||||||
|
current = crd[0]
|
||||||
|
for i in range (1, len(crd) - 1):
|
||||||
|
if crd[i] == "pick_garbage":
|
||||||
|
output.append(99)
|
||||||
|
continue
|
||||||
|
else:
|
||||||
|
output.append(define_dir(current, crd[i]))
|
||||||
|
current = crd[i]
|
||||||
|
|
||||||
|
|
||||||
|
return output
|
||||||
|
|
||||||
|
def save_moveset(moveset, maps):
|
||||||
|
|
||||||
if platform.system() == 'Windows':
|
if platform.system() == 'Windows':
|
||||||
path = '\moveset_data.json'
|
path = '\moveset_data.json'
|
||||||
else:
|
else:
|
||||||
@ -38,7 +76,6 @@ def save_moveset(moveset):
|
|||||||
open(output_file, 'a').close()
|
open(output_file, 'a').close()
|
||||||
finally:
|
finally:
|
||||||
f = open(output_file, 'r+')
|
f = open(output_file, 'r+')
|
||||||
|
|
||||||
try:
|
try:
|
||||||
results = json.load(f)
|
results = json.load(f)
|
||||||
except:
|
except:
|
||||||
@ -47,7 +84,18 @@ def save_moveset(moveset):
|
|||||||
if "moveset" not in results:
|
if "moveset" not in results:
|
||||||
results = { "moveset": [] }
|
results = { "moveset": [] }
|
||||||
|
|
||||||
results["moveset"].append(moveset)
|
dirs = parse_coords(moveset)
|
||||||
|
|
||||||
|
while(True):
|
||||||
|
try:
|
||||||
|
ind = dirs.index(-1)
|
||||||
|
dirs.pop(ind)
|
||||||
|
maps.pop(ind)
|
||||||
|
except:
|
||||||
|
break
|
||||||
|
|
||||||
|
results["moveset"].append({'maps': maps[:-2], 'moves': dirs})
|
||||||
f.seek(0)
|
f.seek(0)
|
||||||
json.dump(results, f, indent=1)
|
json.dump(results, f, indent=1)
|
||||||
f.close()
|
f.close()
|
||||||
|
|
||||||
|
Loading…
Reference in New Issue
Block a user